Julia
This is a list of resources and articles about the Julia language.
Jump to: Documentation • Libraries • Other
News
25/4/2025 18:02 My Interview With Dr. Lee Phillips Regarding His Science Career, Science Writing, and Telling Science Stories: My chat at the National Portrait Gallery in Washington, DC, with Dr. Anwar Dunbar (previously released in six parts, now collected together).
17/02/2025 22:19 Web Applications with Julia: My article appeared in the February edition of “ADMIN magazine”. You can buy the issue or single articles at the link, or pick up the magazine at many bookstores.
15/2/2025 16:51 Asynchronous programming in Julia: Deep and detailed study in the form of a notebook.
5/2/2025 8:06 Julia & JuliaHub: Advancing Innovation and Growth: Brief summary of progress in Julia adoption since 2020.
31/01/2025 13:28 New horizons for Julia: My article about Julia 1.11 and new directions for the language. I talk about new tooling for installation, compilation to small binaries, Julia in browsers, and more.
8/11/2024 17:26 Tensorial: “Tensorial.jl provides statically sized Tensor type that is compatible with AbstractArray, similar to SArray from StaticArrays.jl. In addition to supporting basic AbstractArray operations, the package offers a tensorial interface and several advanced features”.
03/11/2024 09:34 2D Vortex Dynamics: Evolution of a vortex system in two dimensions. An interactive webpage using Julia.
10/10/2024 11:38 Julia 1.11 Highlights: The new release contains some significant developments and enhancements.
22/9/2024 17:11 Practical Julia Errata Page: I’ve added a list of errata and other notes to the book’s website supplement.
10/9/2024 15:10 DBCollections.jl: Lets you “use the exact same code to manipulate regular Julia collections and SQL databases.” This will be immediately useful: data persistence, using arrays not limited by RAM, and more.
30/8/2024 10:35 MicroGrad.jl: Part 1 ChainRules: An extensive tutorial on automatic differentiation in Julia in the context of machine learning.
29/8/2024 22:57 New symbolic solver for Symbolics.jl: This notice in the Julia Discourse discussion group announces the merge of a new solver that significantly increases Symbolics’ capabilities.
29/8/2024 22:36 A new non-parametric method to infer galaxy cluster masses from weak lensing: “a new, non-parametric method to infer deprojected 3D mass profiles M(r) of galaxy clusters from weak gravitational lensing observations…We provide an efficient implementation in Julia code that runs in a few milliseconds per galaxy cluster.”
12/8/2024 12:59 Fermions.jl: New Julia physics library: “Fermions.jl is a toolkit for designing and analysing second-quantised many-particle Hamiltonians of electrons, potentially interacting with each other.”
29/7/2024 11:00 GeoStats.jl: “An extensible framework for geospatial data science and geostatistical modeling fully written in Julia.” Also see the book.
29/7/2024 10:53 Geospatial Data Science with Julia: “best practices for writing clean, readable and performant code in geoscientific applications involving sophisticated representations of the (sub)surface of the Earth such as unstructured meshes made of 2D and 3D geometries.” Open source and CC licensed.
26/7/2024 17:28 waterlily.jl: “A differentiable and backend-agnostic Julia solver to simulate incompressible viscous flow and dynamic bodies”.
23/7/2024 23:23 Diagramming and Data Visualization with Julia: I didn’t know about Vizagrams.jl, an interesting Julia library for plotting and data visualization. It implements a kind of grammar of graphics, and looks powerful.
Documentation and tutorials
StaticCompiler - Generating small binaries: Useful notes on using StaticCompiler to generate small binaries from Julia code.
Using the FFTW Library in Julia: A useful tutorial.
PrecompileTools.jl: Documentation and tutorials for PrecompileTools.jl, which “is designed to help reduce delay on first usage of Julia code. It can force precompilation of specific workloads…You can use PrecompileTools as a package developer, to reduce the latency experienced by users of your package for ‘typical’ workloads; you can also use PrecompileTools as a user, creating custom ‘Startup’ package(s) that precompile workloads important for your work.”
IdSet in Julia 1.11: A new public type in Julia 1.11 and what it’s good for. As a bonus, a useful summary of Julia’s various equality tests.
Storing vectors of vectors in DataFrames.jl: Extremely useful tutorial on preventing vectors from being expanded into multiple rows when applying transformations to dataframes.
Pkg.jl and Julia Environments for Beginners: Exceptionally clear tutorial about Julia’s package manager.
Mastering Efficient Array Operations with StaticArrays.jl in Julia: Good tutorial with benchmarks.
My understanding of object property access in Julia: Clarifies the use of fields and properties of composite objects.
DataFrame vs NamedTuple: a comparison: Informative comparison of the use of dataframes vs. named tuples to represent tables in Julia, with interesting examples of fast and slow code.
Setting up your Julia session: A tutorial about the Julia startup file.
Learning to create a vector in Julia: Two ways to turn a multidimensional array into a Vector in Julia: one that makes a copy and one that uses an alias.
Geospatial Data Science with Julia: CC licensed book.
Fundamentals of Numerical Computation: “This is a revision of the textbook Fundamentals of Numerical Computation by Tobin A. Driscoll and Richard J. Braun. The book was originally written for MATLAB, but this resource has been adapted to suit Julia.” Text available free online.
My approach to named tuples in Julia: “I find it more natural to think of a NamedTuple as an anonymous struct, that optionally can be integer-indexed to get access to its components.”
PSA: Thread-local state is no longer recommended: “Any code that relies on a specific threadid staying constant, or on a constant number of threads during execution, is bound to be incorrect.”
GPU Programming: When, Why and How?: This course on using GPUs in general computing contains a section (“High-level language support”) with an overview of Julia and Python.
Comparison of plotting packages: Newcomers (and others!) using Julia can be bewildered by the profusion of options for making plots and visualizations. Chris Rackauckas provides a useful summary of the pros and cons of all the major packages.
Filtering grouped data frames in DataFrames.jl: A helpful tutorial by Bogumił Kamiński.
Ref ref: “Today I want to discuss the Ref type defined in Base Julia. The reason is that it is often used in practice, but it is not immediately obvious what the design behind Ref is.”
Guide for writing shell scripts in Julia: From 2019, but may still be useful.
Practical guide: how to contribute to open source Julia projects: This is helpful for those interested in making their first contribution to the ecosystem.
What does it mean that a data frame is a collection of rows?: The main developer of Dataframes.jl explains its interface in this illuminating article.
Julia: The Greeks and Math operators at the command line via LaTeX: If you haven’t tried Julia, this may whet your appetite.
Python Criticism from a Julia Perspective: Contains, among other interesting nuggets, a description of a Julia REPL facility for discovering a list of methods that can act on a given combination of types (I had no idea).
Successful Static Compilation of Julia Code for use in Production: “a successful experiment to statically compile a piece of Julia code into a small
.so
library on Linux, which is then loaded from Python and used in training of a deep learning model.”
Parallel Computing and Scientific Machine Learning (SciML): Methods and Applications: “This book is a compilation of lecture notes from the MIT Course 18.337J/6.338J: Parallel Computing and Scientific Machine Learning…a live document, updating to continuously add the latest details on methods from the field of scientific machine learning and the latest techniques for high-performance computing.”
Metadata in DataFrames.jl: why and how?: Valuable and detailed introduction to this useful feature of DataFrames.jl, by one of its developers and the author of Julia for Data Analysis.
Using Julia on the HPC: A survey of simple examples of using Julia in HPC contexts.
Functional One-Liners in Julia: Introduction to currying used in conjunction with broadcasting, with examples.
Bayesian Estimation of Differential Equations: Tutorials showing how to use Turing.jl to estimate parameters of ODEs from data.
Triangle frenzy: A case study using CUDA for some graphics calculations.
Differentiation for Hackers: “The goal of this handbook is to demystify algorithmic differentiation, the tool that underlies modern machine learning.”
Signal Processing with Julia Language: A tutorial series.
Julia macros for beginners: A clear introduction.
PlutoUI Demonstration: A live demonstration of PlutoUI controls, served with the PlutoSliderServer.
Type-Dispatch Design: Post Object-Oriented Programming for Julia: “I am going to try to explain in detail the type-dispatch design which is used in Julian software architectures. It’s modeled after the design of many different packages and Julia Base […] Julia is built around types. Software architectures in Julia are built around good use of the type system. This makes it easy to build generic code which works over a large range of types and gets good performance. The result is high-performance code that has many features. In fact, with generic typing, your code may have more features than you know of! The purpose of this tutorial is to introduce the multiple dispatch designs that allow this to happen.”
Julia Data Science: A free and open-source book in several formats, including a paperback on amazon. Confined mainly to a brief language introduction, dataframes, and plotting with Mackie.
Particle Simulations with Julia: Excellent tutorial and demonstration available as a web page and as a Pluto notebook. Contains fascinating animations of error propagation in a running simulation.
Julia Books: A list from Julia headquarters of works available now or on the way.
What is a Symbol in Julia?: Stefan Karpinski’s masterful explanation of what a Symbol really is.
Writing type-stable Julia code: Using many examples, this tutorial will help you understand how to do what’s in the title.
Convolutions in image processing: Not much about Julia, but a superb demonstration of how simple convolutions can create blurring, sharpening, and edge detection. Ends by showing how to speed up the calculation using FFTs.
HPC Cafe: An introduction to the Julia programming language for HPC: This video is packed with insights about high performance computing with Julia, and is easy to follow.
Julia Meta Types: “One of my favorite tools and a double edged blade in the Julia programming language are Meta Types. Loosely related to Metaprogramming, meta types, derived from the Greek word μετα, ‘transcend’ beyond a simple data structure. Rather than simply describe a physical data structure in memory, a meta type includes information on the type itself.”
Multithreading in Julia Language Applied to Cell Lists Algorithm: “This article teaches you how to use multithreading in Julia Language to parallelize serial algorithms. As a practical example, we explore how to convert the serial Cell Lists algorithm”.
How to Create Software Packages with Julia Language: “This article will teach you how to create an open-source software package in the Julia programming language and develop your package using Git-based workflows.” This tutorial is a detailed and gentle on-ramp to the subject.
Data Science in Julia for Hackers: “This book is currently in a beta version. We are looking forward to getting feedback and criticism”.
Visualize Squirmers: ‘The visualization shown here is based on simulation data generated as part of the work “Modeling a spheroidal microswimmer and cooperative swimming in a narrow slit”.’
Think Julia: How to Think Like a Computer Scientist: “This book is for anyone who wants to learn to program. No formal prior knowledge is required.” Free to read at the link; a print version is also available.
Ditch Excel and Use Julia Data Frames: A really useful tutorial showing how to use Julia to parse and analyse data from a CSV file.
Functional One-Liners in Julia: A clear tutorial with illustrative examples.
Why I Wrote a Julia Programming Book: Julia as a general-purpose language, and as a good choice for teaching.
The Julia Package Manager: A brief introduction to packages, environments, and making your own package.
A quick introduction to data parallelism in Julia: “If you have a large collection of data and have to do similar computations on each element, data parallelism is an easy way to speedup computation using multiple CPUs […] A major hurdle for using data parallelism is that you need to unlearn some habits useful in sequential computation […] In particular, it is important to use libraries that help you describe what to compute rather than how to compute. Practically, it means to use generalized form of map and reduce operations and learn how to express your computation in terms of them. Luckily, if you already know how to write iterator comprehensions, there is not much more to learn for accessing a large class of data parallel computations.”
How do Recipes actually work?: This is a great resource for quickly coming up to speed with plot recipes. It has useful examples and explains how things work under the hood.
Julia for Beginners: “A beginner-friendly guide to the Julia programming language. […] This book engages the reader with fun programming examples involving building and launching a rocket, implementing simple encryption algorithms used by Roman armies, and simulating a mechanical calculator.”
Julia as a cli calculator: Beautifully done and super useful article about using Julia as a calculator for arrays (but contains so much more).
JuliaLang Antipatterns: “An antipattern is a common solution to a problem that over-all makes things worse than they could have been. This blog post aims to highlight a few antipatterns common in Julia code. I suspect a lot of this is due to baggage brought from other languages, where these are not Antipatterns, but are in fact good patterns. This post is to clear things up.”
Six Months With Julia: Parse-time Transpilation in 80 Lines or Less: A case study in applying Julia’s string macros to allow it to read a different language.
Julia: Learn the New Language for Scientific Computing: My introduction to Julia in Linux Format, available for subscribers to the magazine.
Fast as Fortran, Easy as Python: An introduction to Julia in Linux Pro Magazine.
Multi-node Parallelism in Julia on an HPC (XSEDE Comet): “Today I am going to show you how to parallelize your Julia code over some standard HPC interfaces.”
Metaprogramming in Julia: A Jupyter notebook showing some numerical applications of macros.
Julia 1.0 Documentation: The official source.
Julia for Python Programmers: Although Julia is not very close to Python, this might be a useful reference for a Python programmer beginning the upgrade process.
Julia By Example: Learn basic Julia syntax by example, plus four plotting packages and a taste of DataFrames.
Julia Express: Summary of Julia syntax (pdf). Updated: 12Aug2018.
Julia, Matlab, R, Python Cheatsheat: Convenient table comparing (mostly) matrix operations among these languages (left out APL).
Running Shell Commands from Julia: The Julia language has unusually convenient mechanisms for interacting with the shell and gluing code together. Here’s a useful tutorial.
Libraries
GiFlyThroughPaths.jl: Create “fly-throughs” in 3d visualization: “This package makes it easier to create”fly-through” animations of 3d visualizations.” Works with Makie.
Term.jl: “Term.jl is a Julia library for producing styled, beautiful terminal output.” It can also create input widgets.
RCall.jl: Call R packages from within Julia.
ChunkSplitters.jl: “ChunkSplitters.jl makes it easy to split the elements or indices of a collection into chunks…this can be useful in many areas, one of which is multithreading, where we can use chunking to control the number of spawned tasks”.
StructuralVibration.jl: “This Julia package is intended to provide a set of tools for generating vibration data either to validate new numerical methods or to serve as input data for solving inverse problems such as input state estimation via Bayesian filtering, Bayesian regularization, machine learning, etc.”
GitHub - yolhan83/BloodFlowTrixi.jl: This package implements 1D and 2D blood flow models for arterial circulation using Trixi.jl, enabling efficient numerical simulation and analysis.: BloodFlowTrixi.jl is a Julia package that implements one-dimensional (1D) and two-dimensional (2D) blood flow models for arterial circulation.
Welcome to OSCAR: “OSCAR is a new computer algebra system. OSCAR features functions for groups, rings, and fields as well as linear and commutative algebra, number theory, algebraic and polyhedral geometry, and more. It is built upon several well established systems for mathematical research joined via the Julia programming language.”
WebAssemblyCompiler: Compile Julia programs to run in a web browser. Experimental code.
About.jl: Provides the
about
function, which prints attractively formatted information about any object.
OhMyThreads: “OhMyThreads.jl is meant to be a simple, unambitious package that provides user-friendly ways of doing task-based multithreaded calculations in Julia. Most importantly, with a focus on data parallelism, it provides an API of higher-order functions (e.g. tmapreduce) as well as a macro API
@tasks for ... end
(conceptually similar to @threads
).”
Bonito: “Bonito.jl is a pretty simple package allowing you to render HTML and serve it from within Julia and build up a communication bridge with the Browser.”
Oxygen.jl: “Oxygen is a micro-framework built on top of the HTTP.jl library. Breathe easy knowing you can quickly spin up a web server with abstractions you’re already familiar with.” Supports streams and websockets.
Gnuplot.jl: Julia interface to gnuplot: This is a very well-thought-out way to use gnuplot from Julia (you need gnuplot installed), giving you the best of both worlds. For (the excellent) documentation, go to the
docs/src
folder and browse the .md
files, starting with basic.md
.
Handcalcs.jl: “This package supplies macros to generate LaTeX formatted strings from math[e]matical formulas.” For use in Pluto.
Tidier.jl: “Tidier.jl is a data analysis package inspired by R’s tidyverse and crafted specifically for Julia.”
Trixi.jl: “Trixi.jl is a numerical simulation framework for conservation laws written in Julia. A key objective for the framework is to be useful to both scientists and students. Therefore, next to having an extensible design with a fast implementation, Trixi.jl is focused on being easy to use for new or inexperienced users, including the installation and postprocessing procedures.”
SpeedyWeather: “a global spectral atmospheric model with simple physics which is developed as a research playground with an everything-flexible attitude as long as it is speedy…Why another model? You may ask. We believe that most currently available are stiff, difficult to use and extend, and therefore slow down research whereas a modern code in a modern language wouldn’t have to.”
Microstructure.jl: “Microstructure.jl is a Julia package for fast and probabilistic microstructure imaging… under active development…ocuses on tissue microstructure estimation.”
Strided.jl: Make broadcast operations faster.
KittyTerminalImages: If you use the superb Kitty terminal emulator, this package is for you. It lets Julia display images directly in the terminal, using the Kitty protocol (not sixel, etc.).
My Julia Book is in Libraries: giving me a warm feeling.
DynamicQuantities.jl: Type stable units in Julia: Along the lines of Unitful, but storing dimensions as values rather than as parametric types. This leads to greater efficiency in many circumstances.
Merly.jl: Micro framework for web programming in Julia: Seems similar to Oxygen, but hasn’t been updated since about 2020.
Oxygen.jl: A breath of fresh air for programming web apps in Julia: A micro-framework that provides routing and request/response handling.
Algebra of Graphics: Seems to be a powerful approach for data visualization.
ImplicitPlots.jl: Julia package for the plotting of plane curves and surfaces: “Plot plane curves defined by an implicit function f(x,y)=0.”
Pandoc.jl: Pandoc Interface and Types in Julia: Run Pandoc and write Pandoc filters in Julia. Uses the json serialization, so won’t be as fast as Lua filters.
Stipple: A reactive user interface library for use with Genie. Standard use is over a websocket, somewhat similarly to Phoenix Liveview. Seems quite useful for building interactive web applications with Julia, but Unfortunately undocumented, like most Julia packages.
JuliaHEP: High energy physics packages. Includes compatibility layers and partial replacements for ROOT.
PureFun.jl: Immutable containers for Julia.
Yao: “Welcome to Yao, a Flexible, Extensible, Efficient Framework for Quantum Algorithm Design. Yao (幺) is the Chinese character for normalized but not orthogonal.”
Here’s why quantum computing could be the big break for the Julia Language: Introducing Yao.jl, a Julia library for quantum computing.
Introducing Braket.jl - Quantum Computing with Julia: “Braket.jl is a new, experimental package that lets you build quantum circuits and programs in Julia and run them on Amazon Braket.”
SciML: Differentiable Modeling and Simulation Combined with Machine Learning: A new hub for Julia’s SciML documentation.
TikzGraphs: Exploiting the superb TikZ system from Julia to create high-quality graph diagrams (“graph” in the node-edge sense) conveniently.
Swalbe.jl: A lattice Boltzmann solver for thin film hydrodynamics: A Julia program.
PlanetOrbits.jl: Tools for solving and displaying Keplerian orbits for exoplanets.: “The primary use case is mapping Keplerian orbital elements into Cartesian coordinates at different times. A Plots.jl recipe is included for easily plotting orbits.”
SuiteSparseGraphBLAS.jl: An Introduction: “This blog post serves a couple purposes. The first is to introduce Julia users to GraphBLAS, and the features and performance of the
SuiteSparseGraphBLAS.jl
package. The second is an update on new features in versions 0.6 and 0.7 as well as a roadmap for the near future.”
PairPlots.jl: Julia package for making corner plots, the statistical visualization that lets you compare a collection of distributions all at once.
JuliaAstroSim/AstroNbodySim.jl: “Unitful and differentiable gravitational N-body simulation code in Julia”.
PlutoSliderServer.jl: Web server to run just the
@bind
parts of a Pluto.jl notebook.
Changing Physics education with Julia: Interactivity in physics education; why Julia is an ideal language for physics education; applied to teaching dynamical systems and the DynamicalSystems library, of which the author is the maintainer, and his textbook.
GridWorlds.jl: Help! I’m lost in the flatland!: “A package for creating grid world environments for reinforcement learning in Julia.“ Juliacon presentation here.
FunSQL.jl: Julia library for compositional construction of SQL queries’
JuliaSymbolics: “JuliaSymbolics is the Julia organization dedicated to building a fully-featured and high performance Computer Algebra System (CAS) for the Julia programming language.”
TensorCast.jl: It slices, it dices, it splices!: “This package lets you work with multi-dimensional arrays in index notation, by defining a few macros which translate this to broadcasting, permuting, and reducing operations.”
ThreadPools: A set of macros for concurrency. Last updated around 2021; some of its function is provided by the new interactive task feature in Julia 1.9.
Books.jl: Creates books with embedded output from Julia code, using Pandoc.
JuliaFolds/Folds.jl: A unified interface for sequential, threaded, and distributed fold: Powerful, convenient, does what it’s supposed to do.
EmacsVterm.jl: “Better integration of Julia REPL with Emacs vterm terminal.”
Agents.jl: A Julia package for agent-based modeling. Very easy to set up simulations on a variety of spaces, including Open Street Map.
Pluto’s built-in package management: “Starting with version 0.15, Pluto has a built-in package manager, which means:
- Packages are automatically installed when you use import or using.
- Your package environment is stored inside the notebook file. When someone else opens your notebook with Pluto, the exact same package environment will be used, and packages will work on their computer.
ImageClipboard.jl: Copy & Paste images with Julia
Turing.jl: “Bayesian inference with probabilistic programming.”
Bayesian Statistics using Julia and Turing: “Welcome to the repository of tutorials on how to do Bayesian Statistics using Julia and Turing.”
APL.jl: APL in Julia, with all the beautiful characters, and with Julia’s speed.
Downloads.jl: “The Downloads package provides a single function, download, which provides cross-platform, multi-protocol, in-process download functionality implemented with libcurl.” This is now used by the package manager to retrieve packages.
TerminalPager.jl: This lovely package, announced April 19th, 2021, lets you page horizontally and vertically through a dataframe in the REPL, sort of like paging through a file with
less
.
How the Julia language is making it easy for programmers to use GPU capabilities with JuliaGPU: An interview with Tim Besard, a principal contributor to JuliaGPU.
Introduction to GRUtils: “GRUtils is a refactoring of the module jlgr from GR, a graphics package for Julia. The purpose of GRUtils is to provide the main utilities of jlgr in a more ‘Julian’ and modular style, easier to read, and facilitate code contributions by others.”
CSV: A powerful package for dealing with data stored in comma (or any delimiter) separated value files.
Weave.jl: Scientific reports/literate programming for Julia: “Weave is a scientific report generator/literate programming tool for the Julia programming language. It resembles Pweave, knitr, R Markdown, and Sweave.”
VegaLite.jl: Julia bindings to Vega-Lite: “VegaLite.jl is a plotting package for the julia programming language. The package is based on Vega-Lite, which extends a traditional grammar of graphics API into a grammar of interactive graphics.”
Interact.jl: Interactive widgets to play with your Julia code: For use in Jupyter notebooks, the Atom IDE, or on web pages.
Javis: “an easy to use interface written in Julia to create visualizations and animations”.
Distributions Package: “The Distributions package provides a large collection of probabilistic distributions and related functions.”
BioJulia: The bioinformatics infrastructure for the Julia language.
RangeArrays.jl: “Efficient and convenient array data structures where the columns of the arrays are generated (on the fly) by Ranges.”
Einsum.jl: Einstein summation notation in Julia: Incredibly convenient syntax. Also see Tullio.
Announcing Symbolics.jl: A Modern Computer Algebra System for a Modern Language: “We need new Computer Algebra Systems (CAS) for this new era of computing. We need a CAS that dispatches in the multiple ways we think. We need a CAS that scales exponentially like our problems. We need a CAS that integrates with our package ecosystem, letting people extend parts and contribute back to the core library all in one language. We need a modern CAS in a modern language.”
OhMyREPL.jl: “A package that hooks into the Julia REPL and gives it syntax highlighting, bracket highlighting, rainbow brackets and other goodies.”
StatsPlots.jl: Statistical plotting recipes for Plots.jl: A large variety of statistical visualizations, built on Plots.jl.
Reel.jl: computations caught on camera: Easily turn your Plots.jl or other plots into movies in several formats.
PGFPlotsX.jl: Create plots in Julia using the PGFPlots LaTeX package: “PGFPlotsX is a Julia package to generate publication quality figures using the LaTeX library PGFPlots.”
JLD.jl: Saving and loading julia variables while preserving native types: The Julia Data Format is a dialect of HDF5.
Weave.jl - Scientific Reports Using Julia: Weave is a scientific report generator/literate programming tool for Julia. It resembles Pweave, knitr, R Markdown, and Sweave.
Revise.jl: Automatically update function definitions in a running Julia session: “Revise.jl allows you to modify code and use the changes without restarting Julia. With Revise, you can be in the middle of a session and then update packages, switch git branches, and/or edit the source code in the editor of your choice; any changes will typically be incorporated into the very next command you issue from the REPL. This can save you the overhead of restarting Julia, loading packages, and waiting for code to JIT-compile.”
tullio: “Tullio is a very flexible einsum macro. It understands many array operations written in index notation”.
DEC2D.jl: Discrete Exterior Calculus 2D
PipelessPipes.jl - Even More Convenient Piping: “it allows to omit pipe operators, it implicitly assumes first argument piping if not otherwise stated explicitly, it allows for more helpful error highlighting and enables you to interject arbitrary statements into the pipeline for debugging.”
PlutoUI.jl: Convenient Julian wrappers for HTML input controls, for use with Pluto.
Javis.jl: Julia Animations and Visualizations: Animation library, still in early stages of development. An interesting feature is LaTeX support.
Measurements.jl: “Error propagation calculator and library for physical measurements. It supports real and complex numbers with uncertainty, arbitrary precision calculations, operations with arrays, and numerical integration.”
Oceananigans.jl:
“Fast and friendly fluid dynamics on CPUs and GPUs”
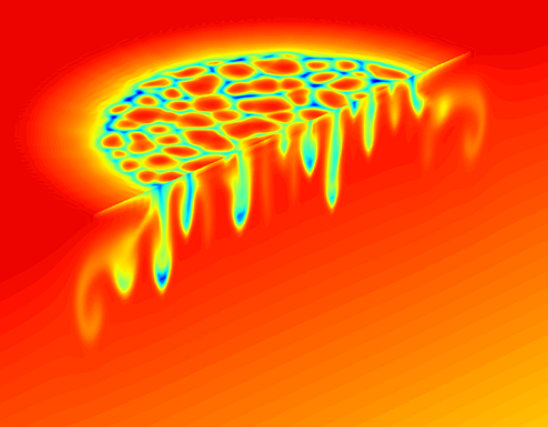
Announcing Gnuplot v1.1.0 - A Julia interface to gnuplot: “the first production ready version of Gnuplot.jl, a package to drive an external gnuplot 16 process from Julia.”
Escher: Webserving with Julia: “With Escher you can build beautiful Web UIs entirely in Julia.” Includes a built-in webserver.
Nemo: A computer algebra package for the Julia programming language.
Gadfly: “Gadfly is a plotting and data visualization system […] influenced heavily by Leland Wilkinson’s book The Grammar of Graphics and Hadley Wickham’s refinment of that grammar in ggplot2.”
PyPlot: An interface to Python’s Matplotlib.
Other
How chaotic is chaos? How some AI for Science / SciML papers are overstating accuracy claims: Instructive and fascinating article by Christopher Rackauckas, the main developer of the amazing Julia package DifferentialEquations. The shadowing lemma is the most interesting thing I’ve learned about this week!
Some SaaS banned me, again: One of the authors of Julia Data Science writes about his bad experiences trying to use Amazon’s Kindle self-publishing platform.
The Plucked String: Simulates the effect of pluck position on the timbre of plucked string instruments. An interactive webpage using Julia.
LibPQ.jl: “LibPQ.jl is a Julia wrapper for the PostgreSQL libpq C library.”
An Interview With Dr. Lee Phillips Part Four: Science Freelance Writing And Practical Julia: We talk about how to break into freelance science writing and about the Julia programming language.
The main thing in Julia 1.11: Bogumił Kamiński describes a new feature: the
@main
macro, which defines an entry point.
Rankings of Julia books on Amazon: Graphs of Amazon’s sales ranks of their books about the Julia programming language.
Julia use in trading leads to massive speedup: Satvik writes on the Julia Discourse: “We use Julia for almost all numeric/data-oriented stuff now, and have moved a lot of the Python code to Julia…At this point 99% of new code I write is Julia, and for the company as a whole it’s about 50-50. The performance differential has become even more ridiculous, an experiment that used to cost ~$125 to run now costs ~.33 cents, and most of those improvements wouldn’t really have been possible in Python.”
Julia use in the development of audio codecs for WhatsApp, Instagram and Messenger.: Video in which engineers from Meta describe how they use Julia to develop advanced audio codecs for their communications applications.
Modern Julia Workflows: “Our purpose is to gather the hidden tips and tricks of Julia development, and make them easily accessible to beginners. We do not cover syntax, and assume that the reader is familiar enough with the basics of Julia. Instead, we focus on all the tools that can make the coding experience more pleasant and efficient.”
Book review: Practical Julia: My book gets a fair and balanced treatment from the preeminent publication covering the free software universe.
New stack traces in Pluto: more colorful, less intimidating!: I recently wrote about the new features in Julia v1.10, including the friendlier, more useful stacktraces in the REPL. Now the amazing Fons van der Plas has released a new version of Pluto, the reactive computational notebook for Julia, with powerful and helpful stacktraces inspired by the new REPL improvements—they take error reporting to a new level.
What’s the deal with Julia binary sizes?: Traces the huge decreases in the sizes of compiled binaries.
Femtolisp: a lightweight, robust, scheme-like lisp implementation: The Julia parser up to Julia v1.10. “This project began with an attempt to write the fastest lisp interpreter I could in under 1000 lines of C.”
Comparing the Performance of Julia on CPUs versus GPUs and Julia-MPI versus Fortran-MPI: a case study with MPAS-Ocean (Version 7.1): “In the end, we were impressed by our experience with Julia. It did fulfill the promise of fast and convenient prototyping, with the ability to eventually run at high speeds on multiple high-performance architectures – after some effort and lessons learned by the developers.”
Julia v1.10 Release Notes: Excellent progress, especially in package loading times. Watch for my LWN writeup, coming soon.
Julia v1.10: Performance, a new parser, and more: My article about the Julia v1.10 release appeared today in LWN.
Julia as a unifying end-to-end workflow language on the Frontier exascale system: “Julia emerges as a compelling high-performance and high-productivity workflow composition language, as measured on the fastest supercomputer in the world.”
Generalizing Scientific Machine Learning and Differentiable Simulation Beyond Continuous models: Data-driven Physical Simulations Seminar Series talk by Chris Rackauckas.
Julia in the Wild: Examples: Case studies of Julia use in science, engineering, industry, and finance.
Practical Julia: My introduction to the best language for scientific computing.
JuliaCon 2023 Live Streaming: Some of the program of this year’s Julia conference will be streamed on YouTube, free to watch.
Potential of the Julia programming language for high energy physics computing: “In this paper the applicability of using the Julia language for HEP research is explored, covering the different aspects that are important for HEP code development: runtime performance, handling of large projects, interface with legacy code, distributed computing, training, and ease of programming. The study shows that the HEP community would benefit from a large scale adoption of this programming language.”
Julia 1.9 Brings More Speed and Convenience: My article about the goodies in Julia v.1.9 appeared today in LWN.
Threadpools in Julia: My favorite new feature in Julia v.1.9: spawn as many tasks as you want, but keep a thread reserved for the REPL, so it continues to respond instantly.
Extreme Multi-Threading: C++ and Julia 1.9 Integration: With Julia v.1.9 we can call Julia libraries from multi-threaded C++ programs, apparently. This article shows you how.
Julia 1.9 Highlights: Julia v.1.9 is now officially released. I’ve been enjoying the 1.9 series since the beta releases. The new version offers significant performance improvements and is even more convenient for development.
Inviscid and incompressible Navier-Stokes equations in 2D implemented in Julia and compiled to WASM: This is quite wonderful and amazing: a Julia fluid solver compiled to WASM and running in the browser. Users can create flow boundaries with the mouse.
Julia for Biologists: “Major computational challenges exist in relation to the collection, curation, processing and analysis of large genomic and imaging datasets, as well as the simulation of larger and more realistic models in systems biology. Here we discuss how a relative newcomer among programming languages—Julia—is poised to meet the current and emerging demands in the computational biosciences and beyond. Speed, flexibility, a thriving package ecosystem and readability are major factors that make high-performance computing and data analysis available to an unprecedented degree. We highlight how Julia’s design is already enabling new ways of analyzing biological data and systems, and we provide a list of resources that can facilitate the transition into Julian computing.”
Solving one based indexing: Finally, a solution to the non-problem of 1-based indexing in Julia that should satisfy everybody.
2021_FortranCon Benchmarks: Comparison of of code and performance between Julia and Fortran on a 2D particle simulation.
Accuracy of tropical peat and non-peat fire forecasts enhanced by simulating hydrology: Fire forecasting using Flux.jl.
Oceananigans.jl: Fast and friendly geophysical fluid dynamics on GPUs: “Oceananigans.jl is a fast and friendly software package for the numerical simulation of incompressible, stratified, rotating fluid flows on CPUs and GPUs. Oceananigans.jl is fast and flexible enough for research yet simple enough for students and first-time programmers. Oceananigans.jl is being developed as part of the Climate Modeling Alliance project for the simulation of small-scale ocean physics at high-resolution that affect the evolution of Earth’s climate.”
Switching from Common Lisp to Julia: “A sufficiently rich parametric type system with multiple dispatch integrated into the language and supported by a JIT compiler is the secret weapon of Julia” and the core reason for the author’s adoption of the language for scientific computing.
C. Brenhin Keller: An assistant Professor of Earth Sciences at Dartmouth using Julia in research and teaching. Many links to Julia Earth science resources.
What’s great about Julia?: The other side of the coin revealed by the author of “What’s bad about Julia?”.
Julia: The Goldilocks language: Contains some interesting details about the origins and history of Julia, along with some odd remarks (“elements of the high-level functionality of MATLAB and R with the speed of C or Ruby”).
Bridging HPC Communities through the Julia Programming Language: “The Julia programming language has evolved into a modern alternative to fill existing gaps in scientific computing and data science applications.”
The best Julia programming books going into 2023: Description of books the author has used plus notes about others, including books arriving in the near future.
The Essential Tools of Scientific Machine Learning: An overview of the state of packages and libraries for scientific ML as of 2019, not confined to Julia.
Julia: Project Workflow: Good ideas about organizing Julia projects, including how to incorporate Pluto notebooks.
Why not: from Common Lisp to Julia: Somewhat of a rebuttal to this.
From Common Lisp to Julia: A personal journey from CL to Julia from a graphics and games programmer. Interesting observations, badly in need of editing.
Switching from Common Lisp to Julia: Although written in the pre-1.0 Julia era, the author penetrates into the core of Julia’s uniqueness: “A sufficiently rich parametric type system with multiple dispatch integrated into the language and supported by a JIT compiler is the secret weapon of Julia.” An informative discussion of scientific computing in CL and Julia.
Review of Interactive Visualization and Plotting with Julia by Zea: A knowledgeable author poorly served by a careless publisher.
Julia 1.8 released: I’ve been using the betas for a while and they’ve been stable and responsive. The highlights in 1.8 are typed non-constant globals and improvements in precompilation, both significant for all users.
Julia REPL ↓ trick: Stefan Karpinski reveals a secret superpower in plain sight:
“After evaluating a command from history in the REPL, you can press ↓ and get the next command after that one, so you can replay a sequence of commands by finding the first one and doing ↓⏎ repeatedly”.
A Look at Communication-Intensive Performance in Julia: “if programmers properly balance the computation to communication ratio, Julia can actually outperform C/MPI in a cluster computing environment.”
Jack Dongarra, who made supercomputers usable, awarded 2021 ACM Turing prize: The creative force behind LINPACK and BLAS says that Julia is a good candidate for the next computing paradigm.